How can I employ diverse types of data in my anticipatory innovation work?
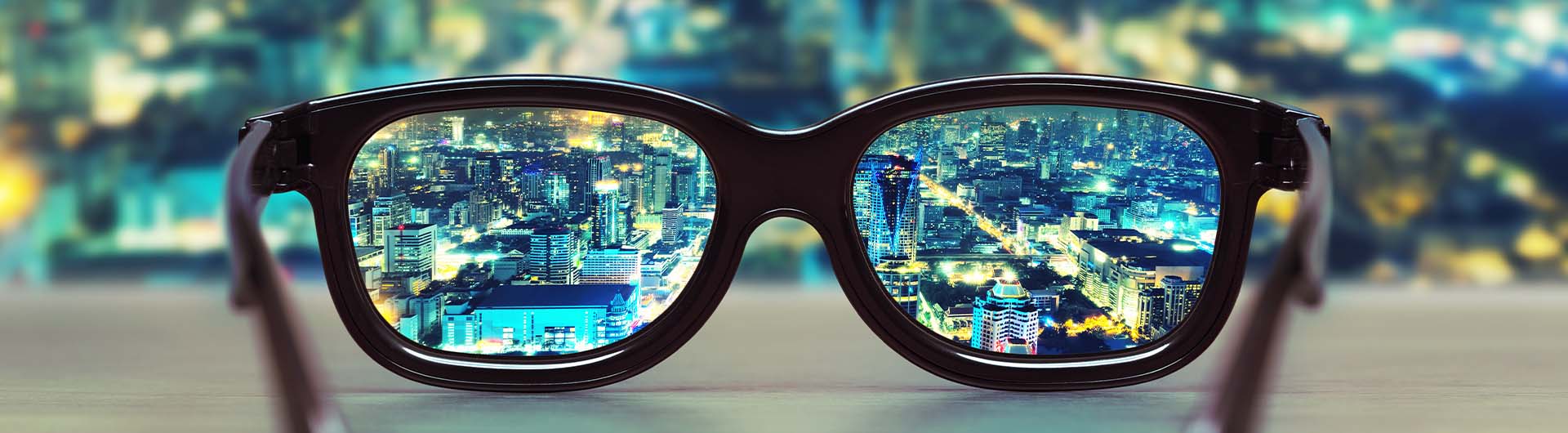
As an interdisciplinary field, anticipation inherently draws on multiple types of data. It is fundamental to any research and intervention design to consider how these different types of data will be employed. In anticipatory processes, there are three stages at which different types of data can be considered for inclusion: input, throughput and output.
At the input stage, different types of data can be considered as indicators or signals of emerging future change. Qualitative data inputs can include literature reviews, expert analysis and interviews, focus group and workshop results, and patent analysis. Quantitative data inputs can include trends, forecasts, text-mining, social media analysis, risk assessments, and quantified indices such as the OECD Services Trade Restrictiveness Index (STRI) or the Programme for International Student Assessment (PISA).
At the throughput stage, different types of data can be used to test coherence and usefulness of anticipatory work, for example by determining whether two phenomena are able to coincide by comparing datasets—such as if certain population numbers could be sustained if water availability is at a given level.
At the output stage, different types of data can be identified and even created as simulations for inspiring initiatives and innovations and as indicators for monitoring their successful implementation. Sometimes the creation or surveillance of data can be the anticipatory innovation itself: starting to gather data for a new indicator that may become more relevant in the future or creating an early warning system for surprise or significant changes are both examples of such innovations.