How can I use anticipation and experimentation as a learning process?
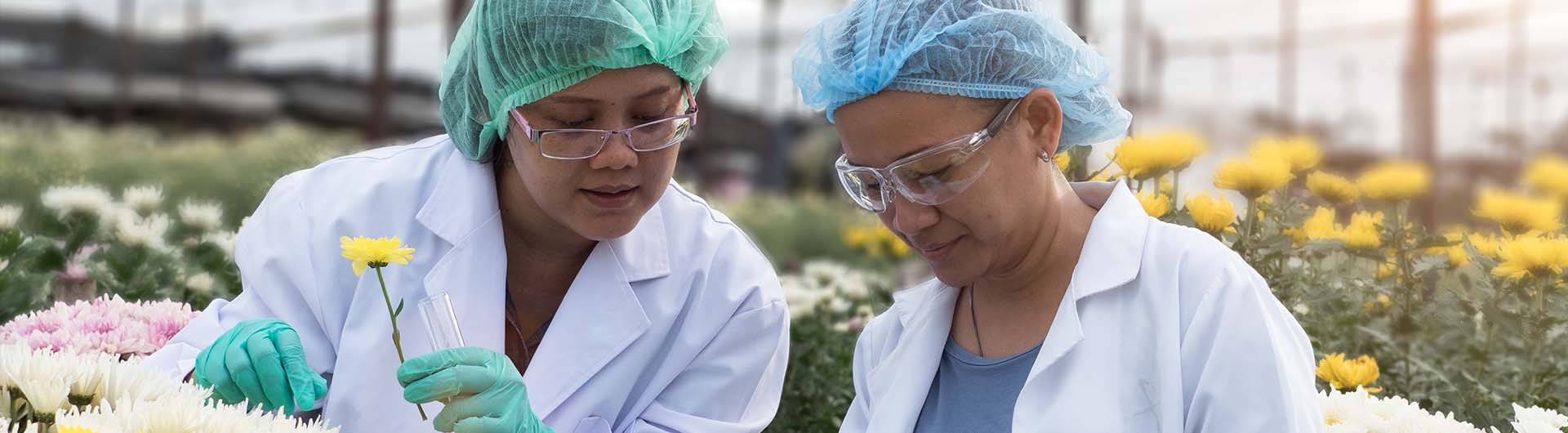
Just as the future is constantly being made and remade, anticipatory innovation is a continuous cycle of anticipating and experimenting: gathering knowledge on possible societal shifts that might manifest in the future, acting on that knowledge in the present and evaluating the results.
Experimentation is central to any innovation process. It can, however, take many different shapes and sizes but is often strongest when approached as a collaborative and iterative process. One useful way of thinking of experimentation is as designing and testing ideas that can be implemented on a small scale first to see how they work out, before considering whether to scale them up—these are known as prototypes. Experimenting through prototypes can help you quickly validate ideas or check assumptions. This is valuable, as it reduces the risk of spending extensive resources and time on potentially going about a problem the wrong way. Some things can only truly be tested at scale, but often times you can learn a lot with much less.
An important question to consider beforehand is whether your experiment is testing a solution or exploring a problem. You can use a prototype to gather data on how a solution works in practice, or you might use it to spark a conversation around the issues you are interested in tackling. This is rarely a clear cut binary distinction but rather a spectrum. It is, however, an important spectrum, as different purposes work better with certain levels of fidelity: If you are validating your approach, a more lifelike prototype might help people to better understand your solution, whereas something more abstract might be better for exploring the problem. This is, in part, due to the fact that the more “real” a prototype looks, the more likely we are to focus on the functionality of it rather than the basic assumptions that underpin it (e.g. “do we need an app for this?”). Designing a prototype ought to be provoking, a way to initiate dialogues that might be hard to otherwise have. One way of doing this is by working with speculative objects that are not designed to provide answers, but rather to ask hypothetical “what-if” questions.
Once you have designed and tested a prototype with relevant stakeholders, it is important to reflect upon the knowledge you have gathered and how it might inform a new version. If your experiment is more involved it’s important to consider what kind of learning mechanisms you build into it: e.g. when and how are you gathering different types of data and how does it feeds back into the experiment.