Artificial Intelligence in the Public Sector – Primer Series #2
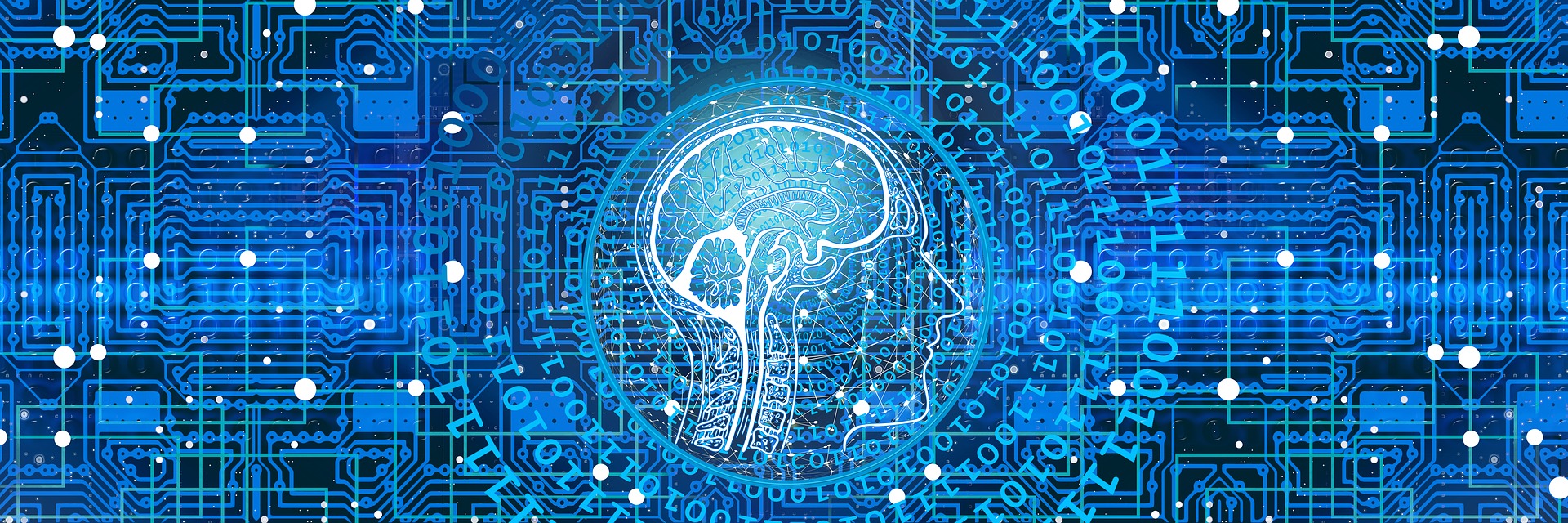
Welcoming your thoughts on our next AI primer
Last year, the OPSI team launched the “Blockchains Unchained” report. This was the first working paper in a series intended to provide the public sector with an overview of the necessary knowledge about a specific emerging technology. The report also aims to help stakeholders understand the challenges and opportunities associated with the technology. To inform public servants and policymakers, OPSI not only draws from academic discussions but also strives to enrich theory with real-world cases (read more on our case study platform) and insights collected through practice (read more about our ongoing projects).
This year, our ambition is to cover Artificial Intelligence (AI) and its impact on the public sector.
AI has been at the centre of attention of many governments all around the world with many countries developing strategies. On the global stage, AI was also the topic of many international events in the past few months: G20, Davos, World Government Summit, and the G7 Multistakeholder Conference on Artificial Intelligence where Canada and France announced the creation of a joint International Panel on Artificial Intelligence (IPAI).
AI is also an issue gaining traction within the OECD, where horizontal teams have integrated AI as part of the Going Digital initiative and are drafting OECD guidelines on AI and our colleagues in the Digital Government team and OECD Working Party of Senior Digital Government Officials (E-Leaders) and the are drafting a working paper on uses of emerging tech (including AI) in governments. Building on these important efforts, we see the need to further explain and explore AI, including sector-specific considerations, limitations, opportunities, and case studies. In order to build upon and support the movement towards higher awareness of AI in the public sector and encourage governments to build capabilities for anticipatory innovation, we are drafting a primer organised around the following questions:
- What is AI?
- What do we mean when we say AI?
- Why talk about it now?
- What role for the public sector in AI?
- What are the different technological approaches (e.g. Machine Learning, Natural Language Processing, Planning-Scheduling-Optimisation, Machine Vision and so on)?
- What are the emerging practices and associated cases?
- How can the public sector act now and prepare for later?
Just as last year’s report was not intended to be an exhaustive, all-encompassing document, the AI primer is all about providing clear and timely knowledge for the public sector audience.
To make sure that we address the most salient points, we invite policymakers, regulators, interested civil servants, and those in industry and civil society to contribute and provide us with comments about the initial structure. We would value as well if there are cases or projects involving AI deemed significant that OPSI could cover and that would bring some light to the public sector. Any other type of inputs would be very much welcomed.
Does our proposed structure cover everything? Is there more that you would like to know? Let us know by adding a comment, emailing is at [email protected], or reaching out on Twitter @OPSIgov by March 22nd, 2019.